Best Data Science Course Training In Hyderabad
The various stages of the Data Science Lifecycle are explored in the trajectory of this course. This Best Data Science Course in Hyderabad begins with an introduction to Statistics, Probability, Python, and R programming. The student will then conceptualize Data Preparation, Data Cleansing, Exploratory Data Analysis, and Data Mining (Supervised and Unsupervised). Comprehend the theory behind Feature Engineering, Feature Extraction, and Feature Selection. Participants will also learn to perform Data Mining(Supervised) with Linear Regression and Predictive Modeling with Multiple Linear Regression Techniques. Data Mining Unsupervised using Clustering, Dimension Reduction, and Association Rules are also dealt with in detail. Best Data Science Course In India
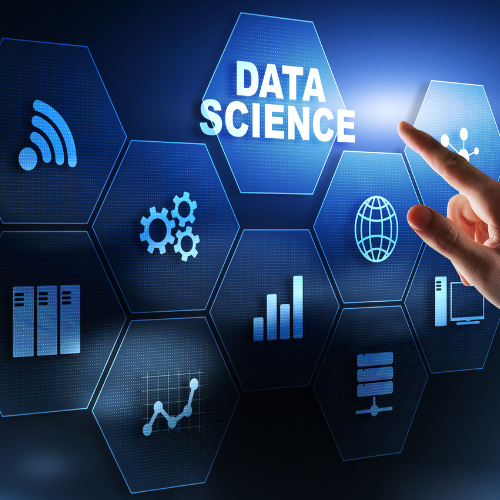
Upcoming Batches
Data Science Course In Hyderabad
Testbug Solutions provides the Data Science Course in Hyderabad to help you become an industry-competent data scientist. The course allows you to learn from scratch and according to the industrial requirements. The Data Science Course has been in high demand in recent years, and our course is designed to provide you with current knowledge in the field. Our approach to practical and theoretical education provides a richer learning experience. Our Data Science institute in Hyderabad is cost-effective and even guarantees an interview at top MNCs and FinTech Startups. Best Data Science Course In India.
Data Science Overview
Data Science Course in Hyderabad Highlights
- Learn the essential fundamentals of Data science.
- High-end training from experts with 12+ years of experience.
- Tailored course curriculum
- Data analysis test cases for practical exposure
- Mock interviews and assessments.
- Verified Data Science course completion certificate.
- 100% placement assistance
- Resume Preparation
- Build recognized credibility in your profile. Data Science Course In Hyderabad
Course
Curruculum
➢ Introduction to
➢ Python
➢ History of Python
➢ Python Installation
➢ IDE’s – Pycharm
➢ Identifiers
➢ Statements
➢ Comments
➢ Variables
➢ Basic Python
➢ Types of Data Types
➢ Integers
➢ Float
➢ Complex
➢ Boolean
➢ String
➢ Operators
➢ Memory ManagIntroduction to
Â
Â
Â
Â
➢ Conditional Statements
➢ Iterative Statements
➢ Interruptive Statements
➢ List
➢ Tuple
➢ Set
➢ Dictionary
➢ Functions
➢ Core Python
➢ Arguments Type
➢ Nested Function
➢ Closure Property
➢ Recursion
➢ Files
➢ Text Files
➢ CSV Files
➢ PDF Files
➢ Oops
➢ Inheritance
➢ Polymorphism
➢ Encapsulation
➢ Abstraction
➢ Lambda Function
➢ Advance Python
➢ Map, Filter, Reduce
➢ Regular Expression
➢ Exception Handling
➢ Serialization
➢ REST API
➢ GIT / GIT HUB
Numpy
➢ What is Numpy
➢ History of Numpy
➢ What is Ndarray
➢ Creating Numpy Array
➢ Array Function
➢ Creating Numpy Array
➢ Array Attributes
➢ Creating Multi-Dimensional
➢ Array
➢ Extracting Data from Arrays
➢ Numpy
➢ Using Indexing
➢ Using Slicing
➢ Boolean Indexing
➢ Random Indexing
➢ Resizing & Reshaping
➢ Transpose
➢ Vector multiplication
➢ Array Attributes
➢ Array Operations
➢ Broadcasting Rules
Â
➢ What is Data Manipulation
➢ What is Pandas
➢ History of Pandas
➢ What is Data Structure
➢ Pandas Data Structure
➢ Series
➢ DataFrame
➢ Creating Series
➢ Creating DataFrame
➢ Extracting Data
➢ Manipulation of Data
➢ Inserting Columns & Rows
➢ Changing Columns & Rows
➢ Pandas
➢Deleting column /
rows Re-indexing
➢Options Customization
➢Indexing & Selecting
➢Date Functionality
➢Identifying Outlier
➢Replace NaN using
➢Deleting using Drop,
➢Dropna
➢Concatenate and Merge
➢Groupby, Pivot Table
and Cross Tab
Â
Â
Â
Â
Â
Â
Databases
➢ What is Database?
➢ Types of Databases?
➢ What is DBMS?
➢ What is RDBMS?
➢ History of RDBMS
➢ SQL Server / MySql
➢ CRUD Operation
➢ Select … Where
➢ Insert
➢ Update
➢ Delete
➢ Joins
➢ Primary & Foreign Keys
➢ Connectivity with
Python
MongoDB
➢ What is NoSQL DB
➢ NoSQL DB and SQL DB
➢ History MongoDB
➢ Features NoSQL
➢ Databases
➢ Create & Drop Database
➢ Create & Drop
➢ Collection
➢ Data Types
➢ Create, Insert, Update,
➢ Delete
➢ Query Document
➢ What is Statistics
➢ Types of Statistics
➢ What is Population
➢ What is Sample
➢ Different Sampling
  Techniques
➢ Statistics Terminology
Descriptive Statistics
➢Central Tendency
Measure
➢Measure of Variability
➢Dispersion Measures
➢Data Distributions
Inferential Statistics
➢ Hypothesis
➢ Types of Hypothesis
➢ Null Hypothesis
➢ Alternative Hypothesis
➢ Chi-Square Test
➢ Anova Test
➢ T-Test
➢ Z-Test
Â
Outlier Detection
➢ Standard Deviation
Method
➢ Inter Quartile Range
Method
➢ Z-Score Method
➢ Percentile Method
Exporatory Data Analysis
➢ Uni – Variate Analysis
➢ Bi – Variate Analysis
➢ Multi – Variate Analysis
➢ Matplotlib
➢ Seaborn
Encoding Techniques
➢ Pandas Dummies
➢ One Hot Encoding
➢ Label Encoding
➢ Ordinal Encoding
➢ Lambda with Apply
Function
➢ Lambda with Map
Function
Inferential Statistics
➢ Hypothesis
➢ Types of Hypothesis
➢ Null Hypothesis
➢ Alternative Hypothesis
➢ Chi-Square Test
➢ Anova Test
➢ T-Test
➢ Z-Test
Imbalance Dataset
➢ Under Sampling
➢ Over Sampling
Matplotlib
➢Bar Graph.
➢Pie Chart.
➢Box Plot.
➢Histogram.
➢Line Chart
➢Subplots
➢Scatter Plot.
Â
Tableau
➢What is Tableau
➢Tableau Architecture
➢Server Components
➢Install Tableau
➢Data Connections to
➢Databases
➢Types of Filters
➢Groups in Tableau
➢Tableau Charts
➢Tableau Graphs
Seaborn
➢Count plot
➢Heatmap
➢Scatter plot
➢Pair plot
➢Violin Plot
➢Box plot
➢Strip Plot
➢Swarm Plot
Â
Â
Supervised Learning
Classification
➢Logistic Regression
➢Decision Tree
➢SVC – SVC
➢Naïve Bayes
➢KNN
➢Ensemble
➢Random Forest
➢Ada Boost
➢GradientBoost
➢XGBoost
Regression
➢Linear Regression
➢Multi Linear Reg
➢Polynomial Reg
➢Lasso Regression
➢Ridge Regression
➢Decision Tree
➢SVM — SVR
➢Ensemble Methods
Unsupervised Learning
Clustering
➢K-Means
➢C-Means
➢Hierarchical
➢Neural Network
➢What is Correlation
➢What is Regression
➢What is Linear
Regression
➢Linear Regression
Overview
➢Simple Linear
Regression
➢Multi Linear Regression
➢Polynomial Regression
➢Related Concepts
➢Bias
➢Variance
➢Bias-Variance Tradeoff
➢Under Fitting Problem
➢Over Fitting Problem
➢What is Regularization
➢Types of Regularization
➢Lasso Regression
➢Ridge Regression
Mathematical Intuition
➢Linear Regression
➢Polynomial Regression
➢Lasso Regression
➢Ridge Regression
Regression / Evolution
Metrics
➢What is Actual Value
➢What is Predicted Value
➢What is Residual
➢R Squared (R^2)
➢Mean Squared Error
(MSE)
➢Root Mean Squared
➢Error (RMSE)
➢Mean Absolute Error
(MAE)
Â
Â
Decision Tree Classifier
➢What is Decision Tree?
➢Terminology of DT
➢Root Node
➢Splitting
➢Decision Node
➢Leaf Node
➢Pruning
➢Sub Algorithm of DT
➢CART Algorithm
➢ID3 Algorithm
➢Gini, Entropy,
Information Grain
➢Mathematical Intuition
➢Real World Data
➢Implementation
Naive Bayes Algorithm
➢What is Probability
➢Conditional Probability
➢What is Bayes Theorem
➢Naïve Bayes Algorithm
➢Types of Naïve Bayes
Logistic Regression
➢Logistic Regression
Overview
➢What is Sigmoid
Function
➢Mathematical Intuition
➢Implementation on real
world data
Support Vector Machines
➢Introduction to SVMs
➢SVC & SVR
➢SVM History
➢Vectors Overview
➢Decision Surfaces
➢Linear SVMs
➢The Kernel Trick
➢Non-Linear SVMs
➢The Kernel SVM
➢Ensemble Learning
➢Weak Learning?
➢Types of Ensemble
Learning
➢Boosting Algorithms
➢Ada Boost
➢GradientBoost
➢XGBoost
➢Implementation of
Ada Boost
➢GradientBoost
➢XGBoost
➢Types of Hyperparameter
Tuning
➢Grid Search Tuning
➢Randomize Search
➢Tuning
➢Why we need Cross
Validation
➢Types of Cross Validation
➢Leave One Out Cross
Validation
➢Hold Out Cross
➢Validation Method
➢K-Fold Cross Validation
Method
➢Stratified Cross Validation
Method
Clustering
➢What is Clustering
➢Types of Clustering
Methods
➢Partitioning Clustering
➢Hierarchical Clustering
➢Density Based
Clustering
➢K-Means Clustering
algorithm
➢Implement K-Means
➢Hierarchical Clustering
Algorithm
➢Implement Hierarchical
Clustering
Image Processing using
Opencv
➢Image to Numpy Array
➢Grayscale Image
➢Image Resize
➢Image Events
➢Image Flip
➢Image crop
Time Series Analysis
➢Time Series data ?
➢Format Time Series data
➢components of Time
➢Series data
➢Time Series scenarios
➢Time Series Model
➢Selection
➢Time Series Model for
  Forecast
➢What is ARIMA Model ?
➢Implementation of
➢ARIMA model
Deep Learning
➢What is Deep Learning
➢Machine Learning VS    DeepLearning
➢Biological NeuralNetwork
➢Deep Learning Application
➢Artificial Neural Network(ANN)
➢Convolutional Neural Network(CNN)
➢Recurrent Neural Network(RNN)
Tensor Flow
➢What is TensorFlow
➢What are Tensors
➢Tensor Graph
➢TensorFlow Perceptron
➢Single Layer
➢Perceptron
➢Hidden Layer
➢Perceptron
➢Multi-Layer Perceptron
Activation Function
➢What is Activation
Function
➢Types of Activation
Function
➢What is Optimizer
➢What is Loss Function
Artifical Neural Network
➢The Detailed ANN
➢How do ANNs work
➢Gradient Descent
➢Stochastic Gradient
 Descent
➢Forward Propagation
➢Backpropagation
➢limitations of a Single
➢Perceptron
➢Neural Networks in Detail
 Understand
➢Backpropagation
Computer Vision (Using CNN)
➢Convolutional Neural
 Network
➢Why CNN
➢Application on CNN
➢Convolutional Layers
➢Pooling Layers
➢Batch Normalization
 Layers
➢Dropout Layers
Natural Language Processing
➢Natural Language
Processing?
➢Tokenization
➢Stemming
➢Lemmatization
➢Stop Words
➢Phrase Matching
➢Vocabulary
➢Part of Speech Tagging
➢Named Entity
➢Recognition
➢Part of Speech Tagging
➢Named Entity
➢Recognition
➢Sentence Segmentation
➢Sentiment Analysis
with NLTK
➢Text Classification
➢Recurrent Neural
Network
Hadoop
➢Hadoop Introduction
➢Hadoop Architecture
➢Hadoop Eco – System
➢HDFS
➢Hadoop Coursera
➢Py-Spark
➢Hive
AWS
➢Cloud Computing
➢AWS Introduction
➢Creating AWS Account
➢EC2 Details
➢Deploying Flask & ML
  Model
Flask
➢Flask Introduction
➢Flask Application
➢Flask URL
➢Templates
➢Merge the ML Model
Kafka
➢What is Message
  Service
➢Kafka Introduction
➢Kafka Architecture
➢Implementation with
  Python
AGILE SCRUM METHODOLOGY
➢Agile Introduction
➢Advantages of Agile
➢Scrum Introduction
➢Scrum Process
➢Scrum Terminology
Â